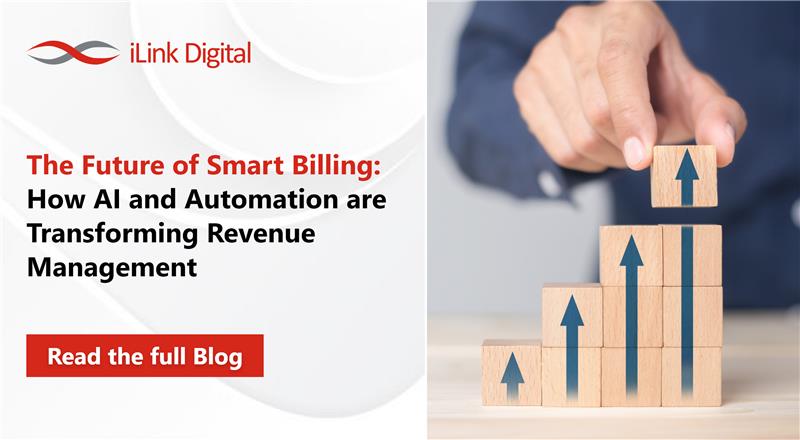
The Future of Smart Billing: How AI and Automation are Transforming Revenue Management
Introduction: Billing as a Strategic Revenue Driver
In today’s hyper-connected digital economy, outdated billing systems are more than just an operational bottleneck—they’re a strategic liability. Manual invoicing, delayed payments, and revenue leakage not only erode profitability but also undermine customer trust. As businesses pivot toward subscription models, global payments, and dynamic pricing, the need for intelligent, agile billing solutions has never been more urgent.
Smart Billing has emerged as a game-changer, powered by Artificial Intelligence (AI), automation, and cloud-based platforms. It offers accuracy, speed, scalability, and improved customer experiences, especially as businesses transition to subscription-based models and complex pricing structures.
This blog explores why Smart Billing is essential, the technologies fueling its growth, and actionable steps for businesses to future-proof their billing systems.
Unlock Smarter Billing—Talk to our experts today!
What Is Smart Billing and Why Does It Matter?
Smart Billing is rapidly becoming essential for modern businesses. Traditional billing system were often manual, error-prone, and slow, struggle to keep up with the demands of today’s digital economy. As organizations adopt subscription-based models, global payments, and dynamic pricing, the complexity of billing increases dramatically. This is where smart billing, powered by AI, automation, and real-time analytics, comes into play.
The urgency for adopting Smart Billing is clear: Gartner reports that 45% of companies suffer revenue leakage due to billing errors, while PwC notes the average billing dispute takes over a month (32 days) to resolve. Furthermore, Salesforce research indicates that 68% of customers are likely to switch providers after multiple billing issues.
The Impact of Inefficient Billing
Many businesses face significant challenges with legacy billing systems:
- Revenue leakage is a widespread problem, with a large portion of companies losing income due to billing errors. This means that money that should be collected is lost because of mistakes in invoicing, missed charges, or process gaps.
- Billing disputes are common, and resolving them can take weeks, tying up resources and delaying cash flow.
- Customer churn increases when clients experience repeated billing issues. In a competitive market, customers are quick to switch providers if they encounter ongoing payment problems.
These pain points collectively drive demand for smarter, more reliable billing solutions that can adapt to evolving business models and customer expectations.
How Smart Billing Addresses These Challenges
Smart billing platforms use AI and automation to:
- Automate invoicing and payment processing, reducing manual errors and speeding up collections.
- Enable usage-based and dynamic pricing, making it easier to offer flexible plans and scale with customer needs.
- Detect fraud and anomalies before they become costly issues, using AI to flag unusual patterns.
- Provide real-time revenue recognition and compliance, ensuring businesses meet regulatory requirements and have up-to-date financial insights
Salesforce & AI: Advancing Smart Billing Capabilities
Intelligent Billing Powered by Einstein AI
Built natively on the Einstein 1 Platform, Revenue Lifecycle Management leverages AI to guide sellers, automate contract management, and optimize pricing and product catalog management. Salesforce’s Einstein AI significantly enhances billing accuracy and efficiency. It proactively identifies and rectifies anomalies before invoices are finalized, predicts payment behaviors with remarkable precision, and recommends optimized billing cycles. This AI-driven approach minimizes disputes, accelerates payments, and improves overall revenue predictability.
Omnichannel Flexibility and Seamless Experiences
Today’s buyers expect to interact and transact across multiple channels—direct sales, partners, online storefronts, and even in-app purchases. Salesforce’s unified product catalog and modular architecture allow businesses to create seamless, personalized buying journeys, no matter where or how customers engage.
Seamless Automation with Salesforce CPQ & Billing
Salesforce CPQ and Billing streamline the entire quote-to-cash process, automating complex workflows. This integration allows for real-time approvals, accurate usage-based billing, and ensures compliance with regulatory standards such as ASC 606 and IFRS 15. By reducing manual touchpoints, organizations improve billing efficiency and significantly shorten revenue cycles.
Predictive Revenue Management with Salesforce Revenue Cloud
Salesforce Revenue Cloud harnesses predictive analytics and automation to provide accurate revenue forecasts, identify customer churn risks proactively, and streamline renewal processes. This capability not only enhances customer retention but also provides valuable insights that guide strategic decision-making around pricing, packaging, and customer engagement.
The Business Impact of Smart Billing
Adopting Smart Billing has several tangible and impactful benefits:
- Enhanced Accuracy: AI-driven automation substantially reduces billing errors.
- Improved Cash Flow: Reduction in Days Sales Outstanding (DSO) by optimizing billing cycles and accelerating payments.
- Reduced Revenue Leakage: Comprehensive analytics and automated controls minimize revenue loss due to errors or fraud.
- Increased Customer Satisfaction: Accurate, timely billing enhances the customer experience and reduces churn.
KPI |
Benefits |
Billing Accuracy |
Achieve near-perfect invoicing precision, with error rates dropping to less than 0.1%, minimizing costly disputes and manual corrections. |
Days Sales Outstanding |
Accelerate cash flow by reducing the average time to collect payments by 35%, enabling healthier working capital and faster reinvestment. |
Revenue Leakage |
Prevent lost revenue by decreasing leakage by 50–75%, ensuring that all billable services are accurately captured and invoiced. |
Customer Satisfaction |
Boost customer loyalty and retention with a 40% increase in satisfaction, thanks to transparent, timely, and error-free billing experiences. |
|
Ready To Overcome Revenue Leakage? Start Your Smart Billing Journey Now!
Emerging Trends in Smart Billing
Looking ahead, the future of Smart Billing promises several innovative advancements:
- Generative AI for Billing Communications: Automated, personalized billing messages that clearly explain charges, reducing confusion and disputes.
- Blockchain for Transparency: Utilizing blockchain technology to ensure billing integrity, transparency, and regulatory compliance through secure, immutable records.
- IoT-Driven Billing Models: Real-time usage tracking enabled by IoT devices, facilitating dynamic pricing models that accurately reflect consumption patterns.
Practical Implementation Steps for Smart Billing:
Transitioning to Smart Billing requires a structured approach:
- Billing Assessment: Evaluate your existing billing processes and identify key pain points.
- AI Integration: Start with implementing AI-driven invoice validation to immediately reduce billing errors.
- Phased Rollout: Gradually integrate comprehensive billing automation tools such as Salesforce Revenue Cloud to ensure a smooth transition and optimized performance.
Businesses adopting Smart Billing solutions typically experience significant returns on investment within a relatively short period, emphasizing the value and urgency of implementation.
Conclusion:
Billing has evolved from a mere administrative function into a strategic driver of organizational growth and customer satisfaction. Embracing Smart Billing technologies such as AI, automation, and predictive analytics is no longer optional—it’s crucial to staying competitive and resilient in a rapidly changing business environment.
Ready to revolutionize your revenue management?